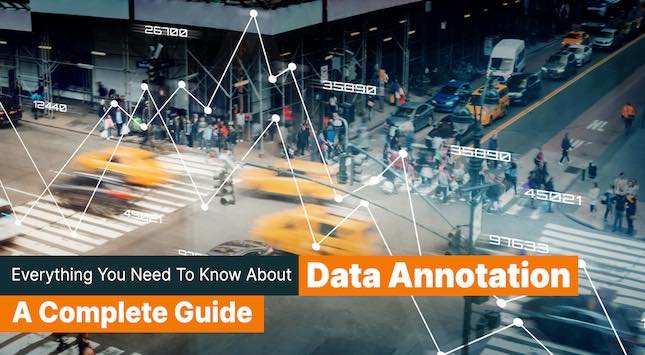
Technologies like artificial intelligence and machine learning have brought about revolutionary changes. They have transformed many possibilities into reality with solutions like self-driving cars, chatbots, high-tech AI security cameras, etc. However, to train such innovative machines & applications you need high-quality, large training datasets. This is where data annotation helps.
Not sure what exactly is data annotation? Read on to know everything about data annotation- what it is, its types, advantages, applications, and more.
What is Data Annotation?
Data annotation refers to labeling data (which could be in any form- text, video, or images) or tagging relevant information in a dataset to make the objects recognizable to computer vision for different ML models.
To make machines perform the desired action & train the machine learning model accurately, data needs to be precisely annotated using advanced tools and techniques. Skilled and experienced data annotator experts access raw data sets and add categories, labels, and other contextual elements, to enable machines to process and act upon the information.
Types of Data Annotation
1. Image Annotation
Image annotation is one of the most critical aspects of computer vision. It refers to the process of labeling digital images that give your computer vision model information about the elements present in the image. The process involves human intervention. However, in some cases, businesses also leverage computer-assisted help.
Either way, images are annotated using different techniques like bounding boxes, polygonal, and semantic annotations and require technical expertise to maintain precision in the outcomes. For that very reason, businesses often go with image annotation services, since such vendors offer a large annotator resource pool as well as enterprise-grade tools.
The image annotation services process is used to train data sets for different models such as autonomous vehicles, face detection, security & surveillance, among others.
2. Video Annotation
Video annotation enables computer vision models to detect & recognize objects in a video. It involves labeling or tagging video clips using human annotators & automated tools. Though it may seem similar to image annotation, the only difference is that video annotation involves annotating video elements on a frame-by-frame basis, thereby making them recognizable for ML models.
Other than simply making objects recognizable to machines, video annotation can also be used for other purposes like studying or processing drone imagery, automated surveillance, object localization, spotting boundaries in video frames, tracking movement, tracking human poses & describing intent, among others.
3. Text Annotation
Text annotation helps machine learning models to understand human communication, i.e., the meaning of sentences, phrases, contexts, and keywords. It is the process of labeling text with additional information or metadata to define the characteristics of sentences, to make them usable for NLP algorithms & machine learning models.
Text annotation services can be applied for various purposes such as designing auto-reply chatbots and conversational AI models, among others. Text annotation processes include three major categories-
- Sentiment annotation- It helps ML models to comprehend the meaning of text beyond directory definitions and make a note of sentiment, and emotional and subjective implications.
- Intent annotation- Under this category, annotators label the intention of the user for machines to understand the context of a conversation and appropriate use of language.
- Semantic text annotation- This involves adding metadata with additional information to annotated text while establishing relevance with the rest of the text.
Data Annotation Applications and Use Cases
Data annotation is being widely accepted across diverse industries. Some of the most common applications & advantages of data annotation are as follows:
Autonomous Driving: With advancing technology, autonomous vehicles will soon become mainstream. However, for cars to develop self-driving capabilities & ADAS features and achieve safety clearance, one needs to train computer-vision-based ML models with high-quality training datasets. To cultivate this capability in AI systems, data annotation practices are used.
AI-based Security Cameras: AI-enabled security cameras provide round-the-clock surveillance and can generate quick alerts if they find any apparent danger, threat, or deceptive activity. However, to function accurately and have precise object detection & face recognition capabilities, the algorithms are adequately trained using data annotation with properly labeled video, image, and text datasets.
Medical & Healthcare: The applications of data annotation in healthcare are paramount. Annotation techniques are widely adopted in the industry for accurate diagnosis. 3D radiological images, MRI, autonomous surgery, radiology, pathology diagnosis, and other advanced imaging systems, use data annotation services to get accurate annotated healthcare datasets to train AI & ML models in the healthcare sector.
eCommerce & Retail: The eStore’s search box is highly optimized with annotated data to show the most accurate results to the users. Furthermore, data annotation is also used for classifying millions of items from various sellers by tagging and sorting products in their relevant categories. Here, AI systems are fed with training data that enables computer vision algorithms to recognize and classify the products while sellers only need to upload the image of the products they want to sell.
Satellite Imagery: Capturing & interpreting the content from satellite imagery uses techniques like object counting, semantic segmentation, object detection, and image classification. Data annotation professionals use high-quality training datasets to accurately annotate images to enable satellites to detect and distinguish between a wide range of elements on the planet. This particular use case of data annotation is specifically beneficial for national security agencies.
Robotics Industry: In robotics, data annotation is used for various aspects like object recognition, object detection in a video, semantic segmentation, sorting products in inventories, predictive maintenance, and much more. The more high-quality training data set is fed to robot models, the more intelligent the & accurate the system will be.
What are the Main Challenges of Data Annotation?
Data annotation is a highly complex, technical, and time-taking job that involves several challenges. Some of the major challenges associated with data annotation are as follows:
Accuracy in annotation: Since the data annotation process and labeling tasks involve human intervention, the outcome is prone to human errors. This can result in poor data quality and hamper the prediction and output of your AI or ML-based application.
High cost of annotation: Data annotation processes require advanced tools, infrastructure, technology, and of course, expert resources. When combined, it costs companies a great deal of money, posing a big challenge for budget management. Furthermore, if developing an in-house team, you will have to pay high salaries to the hired resources since most of them are likely to be certified specialists.
Finding the right annotators: AI/ML projects (especially high-scale) require expert, skilled, and experienced data annotators. Finding the right expertise and building a team from scratch can be highly challenging.
Complex & voluminous data: Training data is often heterogeneous, complex, and voluminous. It is certainly not easy to build capabilities to handle such large amounts of complex data within the company.
AI data collection: AI and ML-based models depend on high-quality data to function. The main challenge for companies is to identify from where to generate massive volumes of quality data. If you do not have the right data to train your ML models, you can bring no value to the application.
Technology and infrastructure: Creating an infrastructure to support the data labeling and annotation project needs a high budget. Furthermore, you need a robust technical infrastructure that involves development, maintenance, and up-gradation. Companies that are not into core technical services often find this overwhelming.
How Do Professional Data Annotation Services Solve These Challenges?
If you are wondering how to deal with challenges associated with data annotation, the best solution lies in outsourcing data annotation services.
Here’s how hiring a professional data annotation company can help-
- Improved accuracy in AI/ML models: Sustained accuracy levels and having a strong quality control is essential to precisely annotate high-volume data and get high-performing results. This is where professional services help. The outsourcing companies use high-quality data to create training data sets and ensure better accuracy and precision while developing your AI/ML-based application.
- Quick turnaround time: Data annotation is a time taking process that needs human intervention and multiple-level accuracy checks. But when you outsource your annotation work to a vendor, they have a dedicated team of data annotators & QA experts who are adept at working on large-level projects thereby ensuring quick delivery of your project, without compromising on the quality.
- Access to advanced tools & technology: As mentioned earlier, data annotation & object labeling tasks involve the use of automated tools and advanced software. Outsourcing companies have access to the latest tools present in the market and can help you make the best out of it.
- Team of highly trained annotators: It is not easy to find experienced and competent data annotators, However, with outsourcing companies, you get access to a full team of highly trained and skilled data annotators who can understand your custom needs and propose the best solutions to meet your specific goals.
- Cost-effective solutions: Since the data annotation process involves heavy costs- including the cost of resources, infrastructure, tools, etc., you can cut down this cost substantially by hiring a professional firm. Outsourcing can cut down the operational cost and save you from unnecessary expenses.
- Flexible hiring models: Outsourcing companies offer flexible and scalable hiring models. This implies that you can hire resources as per your specific requirements- full-time, weekly basis, project basis, etc. You can further scale up or scale down the number of resources as per your customized needs.
Wrapping Up
The application of technologies like artificial intelligence & machine learning will only grow with time, and so will the use of data annotation. With annotation becoming widely applicable & accepted in different industry verticals, you can harness the power of data annotation services for your innovative solutions.
However, building a great application with AL/ML algorithms is possible only when you use high-quality training datasets. Partnering with reliable data annotation services can help you leverage the fine combination of human intelligence and smart tools & create high-quality training data sets for machine learning.